Lantern: PostgreSQL Vector Search Extension
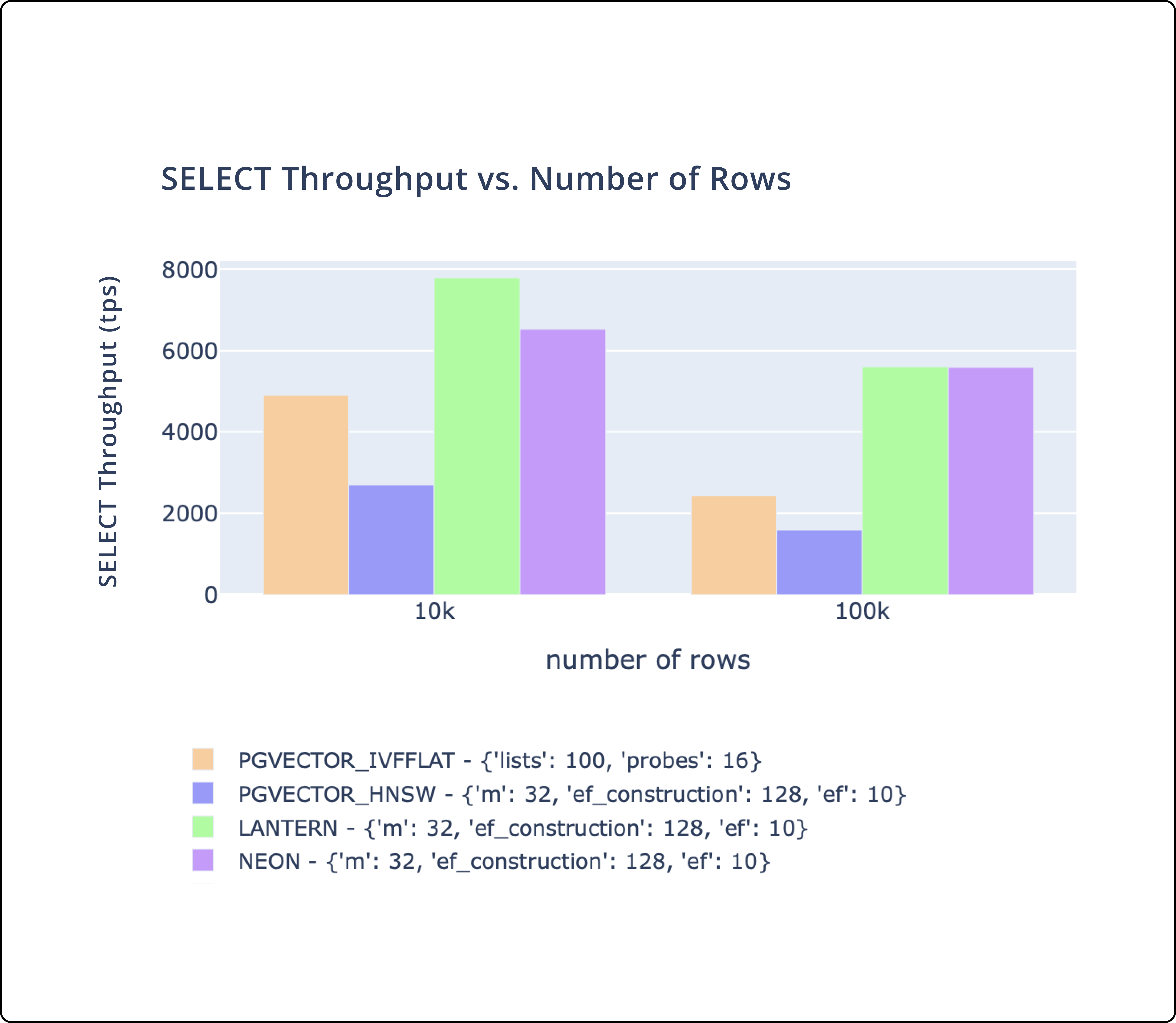
Project Overview
GitHub Stats | Value |
---|---|
Stars | 749 |
Forks | 53 |
Language | C |
Created | 2023-07-11 |
License | Other |
Introduction
Lantern is an open-source PostgreSQL database extension designed to efficiently manage and query vector data. It enables the storage of vector data, generation of embeddings, and execution of vector search operations. A key feature of Lantern is the introduction of a new index type called lantern_hnsw
, which significantly speeds up queries involving ORDER BY ... LIMIT
clauses. By leveraging a state-of-the-art HNSW (Hierarchical Navigable Small World) implementation, Lantern enhances the performance of vector-based queries. This tool is particularly useful for applications requiring fast and accurate vector search capabilities, making it worth exploring for anyone working with large datasets involving vector data.
Key Features
Overview
- Lantern is an open-source PostgreSQL database extension designed to store vector data, generate embeddings, and perform vector search operations.
Main Capabilities
- Vector Data Handling: Supports storing and querying vector data with a new index type called
lantern_hnsw
, which optimizesORDER BY ... LIMIT
queries. - Embedding Generation: Allows generation of embeddings using popular models like CLIP, Hugging Face models, and custom models.
- Indexing: Utilizes the HNSW (Hierarchical Navigable Small World) algorithm for efficient indexing. Supports parallel index creation and external index graph generation.
- Interoperability: Compatible with pgvector’s data type, enabling seamless transition from pgvector to Lantern.
- Performance: Matches or outperforms other solutions like pgvector and pg_embedding in terms of index creation time, select throughput, and select latency.
- Customization: Allows customization of
lantern_hnsw
index parameters such as distance functions, construction parameters, and search parameters.
Installation and Usage
- Can be installed using Docker, Homebrew, or by building from source code.
- Retains the standard PostgreSQL interface, ensuring compatibility with existing tools.
- Supports various distance functions and operator classes for flexible querying.
Additional Features
- Cloud-hosted version planned.
- Future support for hardware-accelerated distance metrics, industry-specific application templates, and more tools for generating embeddings.
- Community contributions and support are encouraged.
Real-World Applications
Vector Search in Database Queries
Lantern can be used to speed up vector search operations in PostgreSQL databases. Here’s an example:
-- Create a table with a vector column
CREATE TABLE small_world (id integer, vector real[3]);
INSERT INTO small_world (id, vector) VALUES (0, '{0,0,0}'), (1, '{0,0,1}');
-- Create an HNSW index on the vector column
CREATE INDEX ON small_world USING lantern_hnsw (vector);
-- Query data using the index
SET enable_seqscan = false;
SELECT id, l2sq_dist(vector, ARRAY[0,0,0]) AS dist
FROM small_world ORDER BY vector <-> ARRAY[0,0,0] LIMIT 1;
Embedding Generation
Lantern supports embedding generation for various models, such as CLIP and Hugging Face models.
-- Generate embeddings using a custom model
-- (Assuming you have a function to generate embeddings)
INSERT INTO embeddings_table (id, embedding)
VALUES (1, generate_embedding('input_text'));
Index Customization
You can customize the lantern_hnsw
index parameters to optimize for your specific use case.
-- Create an HNSW index with custom parameters
CREATE INDEX ON small_world USING lantern_hnsw (vector dist_l2sq_ops)
WITH (M=2, ef_construction=10, ef=4, dim=3);
Exploring and Benefiting from the Repository
-
Quick Installation: Use Docker or Homebrew to quickly set up Lantern with PostgreSQL.
docker run --pull=always --rm -p 5432:5432 -e "POSTGRES_USER=$USER" -e "POSTGRES_PASSWORD=postgres" -v ./lantern_data:/var/lib/postgresql/data lanterndata/lantern:latest-pg15
or
brew tap lanterndata/lantern brew install lantern && lantern_install
-
Build from Source: Build Lantern on top of your existing PostgreSQL installation.
git clone --recursive https://github.com/lanterndata/lantern.git cd lantern mkdir build cd build cmake -DMARCH_NATIVE=ON
Conclusion
Key Points:
- Vector Data Handling: Lantern is an open-source PostgreSQL extension for storing vector data, generating embeddings, and performing vector search operations.
- Performance: It introduces a new index type,
lantern_hnsw
, which speeds upORDER BY ... LIMIT
queries and matches or outperforms existing solutions like pgvector and pg_embedding. - Installation: Easy installation via Docker, Homebrew, or building from source code.
- Compatibility: Retains standard PostgreSQL interface, compatible with existing tools.
- Features: Supports embedding generation, parallel index creation, and external index graph generation.
- Roadmap: Plans include cloud-hosted versions, hardware-accelerated distance metrics, industry-specific templates, and enhanced embedding tools.
Future Potential:
- Performance Improvements: Ongoing enhancements to maintain top performance.
- New Features: Autotuned index types, support for additional vector elements, and version control for embeddings.
- Community Engagement: Encourages community contributions and provides support for troubleshooting and feature requests.
For further insights and to explore the project further, check out the original lanterndata/lantern repository.
Attributions
Content derived from the lanterndata/lantern repository on GitHub. Original materials are licensed under their respective terms.
Stay Updated with the Latest AI & ML Insights
Subscribe to receive curated project highlights and trends delivered straight to your inbox.